2022 PCF – Janssen Special Challenge Award
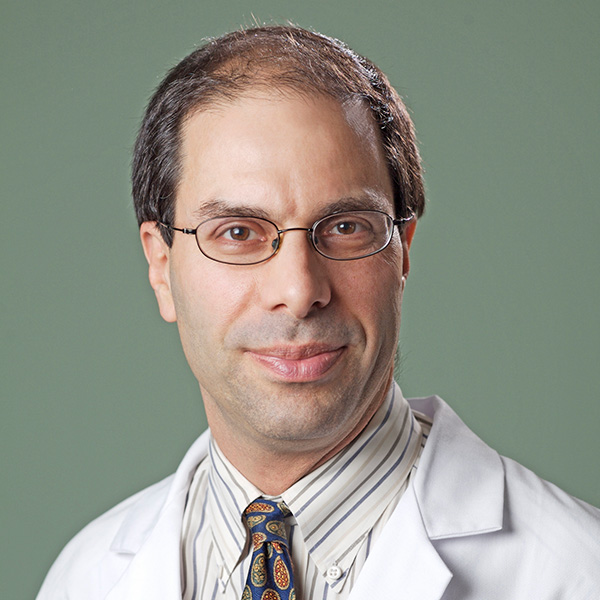
Advancing the Drug Development Process in Metastatic Prostate Cancer through Machine Learning
Principal Investigators: Eric Small, MD (University of California, San Francisco), Rahul Aggarwal, MD (University of California, San Francisco), Julian Hong, MD, MS (University of California, San Francisco), David Quigley, PhD (University of California, San Francisco)
Co-Investigators: Meera Chappidi, MD, MPH (University of California, San Francisco), Adam Foye, BS (University of California, San Francisco), Li Zhang, PhD (University of California, San Francisco)
Description:
- Clinical trials are necessary to test the efficacy and safety of new treatments and provide the data necessary for regulatory approval for patients. However, trials can often take a long time, due in part to the endpoints measured, such as overall survival (OS). This endpoint takes many years to reach in men with metastatic prostate cancer.
- Attempts to identify intermediate biomarkers for overall survival have largely been unsuccessful, as traditional one-dimensional approaches have evaluated simple kinetics or just one or two clinical parameters at a time.
- Eric Small and team are using longitudinal machine learning approaches that can test billions of combinations of kinetic clinical variables, to identify novel patterns that may serve as intermediate endpoints that are highly predictive of overall survival.
- The team will apply machine learning models to comprehensive molecular, clinical, and outcomes data from nearly 6,000 patients with metastatic prostate cancer on clinical trials. Promising models will be validated in large real-world datasets.
- This project will develop and validate two types of models: 1) predicting survival at 72 months, using data from the first 18 months of treatment, and 2) predicting survival at 12 months with data from the first 2 months of therapy.
- If successful, this project will identify intermediate clinical endpoints are highly predictive of overall survival in patients with metastatic prostate cancer and may help to speed clinical trials in this setting.
What this means to patients: The identification of intermediate clinical endpoints which can be used much earlier in clinical trials to determine the impact of a new treatment on patients’ overall survival will greatly speed the development of new treatments. Dr. Small and team will use a machine learning approach to identify novel kinetic, multi-dimensional biomarkers that can serve as accurate predictors of overall survival, which will accelerate the “readout” timeline for late phase studies in metastatic prostate cancer, and lead to faster patient access to effective new therapies.