2024 Michael and Lori Milken Family Foundation-PCF Young Investigator Award
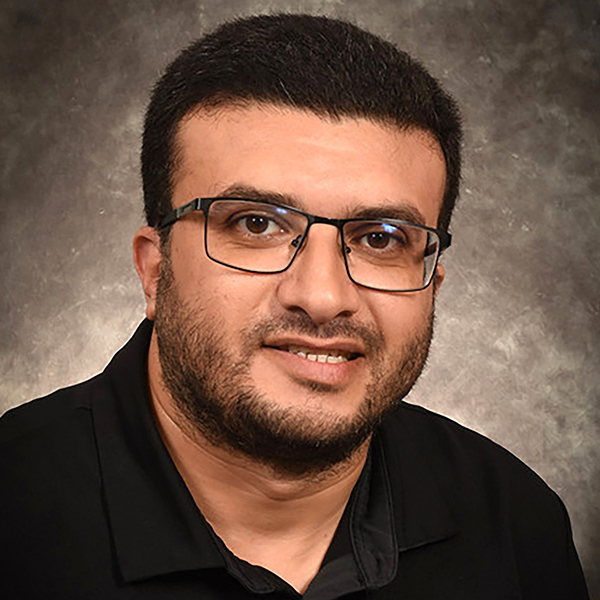
Using Machine Learning to Predict RNA Pol II Interactions of Metastatic Prostate Cancer
Ahmed Abbas Elmahdi, PhD
University of Texas Southwestern Medical Center
Mentors: Ram Mani, PhD; Michael Zhang, PhD
Description:
- Prostate cancer is characterized by genomic rearrangements and structural variations that result in highly dysregulated gene expression programs which drive cancer development and progression.
- Understanding the 3D genomic architecture of prostate cancer is key to understanding disease mechanisms and developing improved treatment strategies.
- Dr. Ahmed Abbas Elmahdi is developing a machine learning-based model to predict the 3D structure of prostate cancer using genomic sequencing data from methods (RNA-seq and ATAC-seq) that are less costly and more feasible than 3D sequencing methods (ChIA-PET).
- This machine learning model will be used to predict the 3D genomic organization of different subtypes of metastatic castration-resistant prostate cancer (mCRPC), including androgen-dependent (AR+) CRPC, and the highly aggressive neuroendocrine prostate cancer (NEPC) and double-negative (AR-/NE-) subtypes.
- This data will be used to identify the gene enhancers that are enriched in different mCRPC subtypes, to identify transcription factors likely to be driving disease.
- The presence of cancer risk genetic polymorphisms in active gene enhancers that may have a role in driving prostate cancer will be investigated.
- If successful, this project will develop and validate a feasible and inexpensive machine learning-based method to model cancer 3D genomics, and will identify transcription factors driving different subtypes of mCRPC that may serve as potential therapeutic targets.
What this means to patients: The 3D genomic organization of cells is a major regulator of gene expression and cellular functions. Understanding how the 3D genome is altered in cancer is key to understanding disease biology and improving therapies. In this project, Dr. Abbas Elmahdi will develop a novel machine learning-based method to model the 3D genome using broadly available genomics sequencing data. This method will be used to model the 3D genome of mCRPC subtypes and predict key drivers that may have potential as therapeutic targets